DFG Research Impulse - Smart Factory Grids
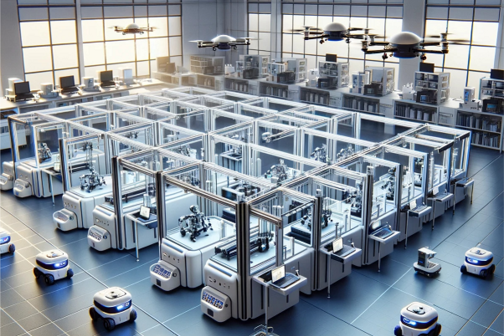
DFG Research Impulse - Smart Factory Grids (2024-2029)
The research initiative "Smart Factory Grids," funded by the Deutsche Forschungsgemeinschaft (DFG, German Research Foundation), focuses on the concept of an intelligent factory. Our vision is a distributed manufacturing network, where multiple specialized production units are interconnected by autonomous (ground-based and aerial) systems to achieve highly flexible manufacturing for small batch sizes, with dramatically reduced setup times. At the heart of these Smart Factory Grids are cyber-physical systems, designed to operate autonomously and cooperatively. These systems will self-assess and adapt, maintaining resilience against disruptions. This is more than just automation; it's about creating a manufacturing ecosystem that's intelligent, responsive, and sustainable.
Project Duration: | 04/2024 - 03/2029 |
Project Team (IIS): | Prof. Dr. Markus Enzweiler + PhD students |
Further information:
Press release (in German)
AnoMoB
Anonymized Collection and Use of Mobility and Movement Data (2022-2025)
For years, new forms of individual and collective mobility have been evolving. Service providers, including car-sharing and public transport, require insights from mobility data to tailor their services to user needs while conserving resources and protecting the environment. The "Anonymized Collection and Use of Mobility and Movement Data" (AnoMoB) project aims to develop anonymization techniques that allow providers to enhance mobility data collection and processing, while safeguarding citizen privacy.
Initially, the project will determine specific data needs of service providers, such as types of mobility data and attributes like age and income, required for planning resource-efficient, user-centric mobility. Based on these requirements, tailored technical solutions will be developed for privacy-compliant data collection, ensuring sensitive information is secured with robust protection measures. This approach also aims to boost user trust in service providers through reliable anonymization of their personal data.
Project Duration: | 12/2022 - 12/2025 |
Project Team (IIS): |
Funded by the Bundesministerium für Bildung und Forschung
HE-Personal
Overview (2021-2027)
Under the joint "FH-Personal" program by the federal and state governments, 64 universities of applied sciences, including Esslingen University of Applied Sciences, are receiving support for recruiting and developing professorial staff. Esslingen University, one of 13 universities in Baden-Württemberg awarded in the first funding round, is set to receive over six million euros for the project. This funding aims to nurture young academics at the university, preparing them as qualified candidates for future professorships at universities of applied sciences (HAW). The "HE-Personal" project at Esslingen University focuses on attracting top-tier academics with practical experience to teach and conduct research across eight forward-looking project clusters.
Project Cluster Autonomous Systems
Autonomous systems, a cornerstone of intelligent robotics, represent one of the most dynamic research domains globally, especially in machine learning applications. This area holds significant strategic value for the Stuttgart region, given its strong industrial base. Within the HE-Personal framework, the emphasis is on artificial intelligence research, particularly in developing methods to minimize resource consumption in AI system development, contributing to the concept of "Green AI". This is crucial as the training of a single artificial neural network can incur substantial costs.
Research topics cover various sub-topics, such as the automation of the architecture of AI systems with a view to increasing efficiency; the learning ability of AI systems, especially from smaller amounts of data, in order to reduce the effort involved in (manual) data collection (self-supervised learning, weak supervised learning, few-shot learning, low-resource NLP); and the acquisition of a deeper understanding of AI systems in order to enable more targeted optimization of the AI architecture (explainable AI).
Project Duration: | 07/2021 - 03/2027 |
Project Team (IIS): | Sophie Böttcher, Prof. Dr. Alexander Brandt, Prof. Dr. Gabriele Gühring, Prof. Dr. Markus Enzweiler |
Project Partners: | University of Tübingen, Robert Bosch GmbH |
Further information: HE-Personal project webpage
Funded by the Bundesministerium für Bildung und Forschung and the Ministerium für Wirtschaft, Arbeit und Tourismus Baden-Württemberg.
Short-Term Wind Energy Forecasting
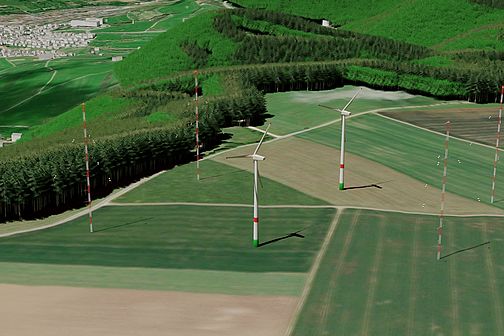
Development of a Self-Learning Method for Improved Short-Term Wind Energy Forecasting (2022-2025)
Using spatially high-resolution, weather model-coupled CFD (Computational Fluid Dynamics) models based on the Reynolds-averaged Navier-Stokes equations (RANS), a high degree of accuracy in predicting wind speed has been achieved. This accuracy is crucial for assessing wind yields at potential sites. This success opens up the possibility of further developing these methods to create short-term forecasts for wind energy yields in complex terrains for wind turbines. The existing approach, which combines mesoscale weather models with artificial intelligence methods for forecasting (hybrid methods), will be enhanced. The focus will be on physical downscaling using detailed CFD simulations and the application of ensemble weather forecasts to determine the physical uncertainty in these forecasts.
Project Duration: | 09/2022 - 09/2025 |
Project Team (IIS): | Götz Grimmer, Prof. Dr. Steffen Schober |
Project Partners: | WEPROG GmbH |
Funded by the Deutsche Bundesstiftung Umwelt (DBU).
Mobile Robotics

Autonomous Robots in Unstructured Environments (2022-)
The Mobile Robotics research project is dedicated to exploring innovative methods enabling mobile robots to operate autonomously in unknown, unstructured environments. Emphasizing sensor-based perception and self-localization, the project leverages cutting-edge machine learning techniques.
In its initial phase, the project involved a thorough analysis and evaluation of existing methods, primarily focusing on robotics in known and structured contexts, such as indoor logistics in industrial settings or autonomous road traffic navigation. These methods, however, proved to be less applicable to uncharted and unstructured environments.
The current project phase delves into adapting these existing methods for more complex, unstructured environments. This involves a comprehensive derivation of new requirements and the creation of a sophisticated system for evaluation and comparison. This system will not only encompass theoretical analyses but also include experimental studies using publicly available research datasets.
Project Duration: | Since 12/2022 |
Project Team (IIS): | Fabian Schmidt, Constantin Blessing, Joshua Uhl, Maxim Becht, Tobias Mitterfellner, Prof. Dr. Markus Enzweiler |
AMEISE
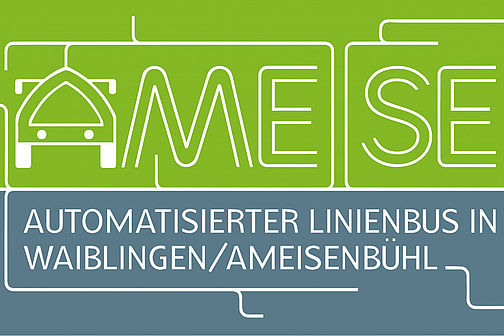
AMEISE (Phase III) - Holistic Research Into the Potential of Autonomous Driving in Public Transport (2023-2024)
In the fall of 2023, the emission-free and autonomous shuttle AMEISE progressed into its third phase of funding. This phase continues to explore how the shuttle can be seamlessly integrated with urban development and engage all relevant stakeholders. The focus is on identifying key factors that will drive the success of this initiative, leveraging expertise to optimize the shuttle's role in the urban landscape.
Project Duration: | 09/2023 - 10/2024 |
Project Team (IIS): | Marcel Voßhans, Alexander Baumann, Götz Grimmer, Prof. Dr. Steffen Schober, Prof. Dr. Clemens Klöck, Prof. Dr. Ralf Wörner |
Project Partners: | See the AMEISE webpage |
Further information: AMEISE webpage
HQ-Forecast
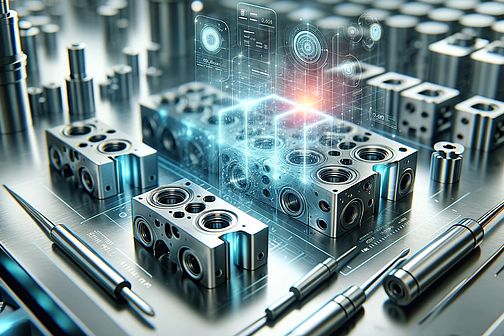
AI-Based Quality Prediction of Injection Molded Parts (2022-2024)
This project's goal is to develop an AI-based forecasting system for predicting the quality of parts in injection molding.
Unlike current technology, this system is designed to significantly surpass existing prediction methods that rely on process monitoring through machine parameters. Furthermore, it aims to require significantly fewer training data than conventional AI applications, making it attractive for small-batch production.
The prediction model developed in this research project is intended to monitor the parts with such accuracy during the production process that post-production quality control becomes redundant.
To achieve this, appropriate sensors will first be integrated into the process. A multi-stage evaluation system allows for rapid deployment of the system, as it requires only a small amount of training data. The multi-stage classification enables the identification of unknown process states and their inclusion during ongoing production. Known process states can be robustly and securely classified by the system using machine learning methods.
Project Duration: | 08/2022 - 07/2024 |
Project Team (IIS): | |
Project Partners: | Feinwerktechnik Otto Harrandt GmbH PlastSolutions GmbH |
Funded by the Ministerium für Wirtschaft, Arbeit und Tourismus Baden-Württemberg.